Ultra high field MRI
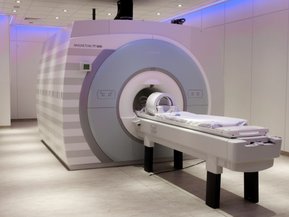
The CBClab’s main measurement modality is ultra-high field (UHF; 7Tesla and above) magnetic resonance imaging (MRI). We are housed adjacent to and help operate the Scannexus UHF MRI facility, with its human 3T, 7T and 9.4T MRI systems. These systems represent the state-of-the-art not only in main magnet strength, but also in gradient and RF-coil technology. Our 9.4T system (83cm bore, 80mT/m high slew-rate head gradient, 8 transmit & 32 recieve RF channels) is one of only about 5 such systems in the world. The main MRI modalities we use to study human brain connectivity are diffusion MRI and functional MRI.
Diffusion MRI (dMRI) probes the hindrance and restriction of diffusing water and is sensitive to the microstructure of biological tissue. In particular, it can be used to study the orientation and properties of neurites in white matter and, at high enough spatial resolution, gray matter in the brain. We specialize in diffusion imaging in post-mortem tissue, which can be performed at very high spatial resolutions (in the 100’s of microns) impossible to attain in vivo. This makes it possible to investigate the mesoscale network architecture of human white matter and gray matter over large parts of the brain, or even the entire human brain.
Functional MRI (fMRI) probes the highly localized changes in oxygenation of blood following neuronal activity in the brain (termed the blood oxygenation level dependent or BOLD contrast mechanism). Since the BOLD fMRI signal is based on the magnetic susceptibility of hemoglobin, its signal and contrast improves drastically with higher main fields. This makes it possible to image the functional activity of the human brain at resolutions below the millimeter at fields of 7T and above. We specialize in functional and causal connectivity analysis of fMRI data. This involves analysis and modeling fMRI signal to uncover interactivng networks and directions of information flow in the brain.
Light Microscopy
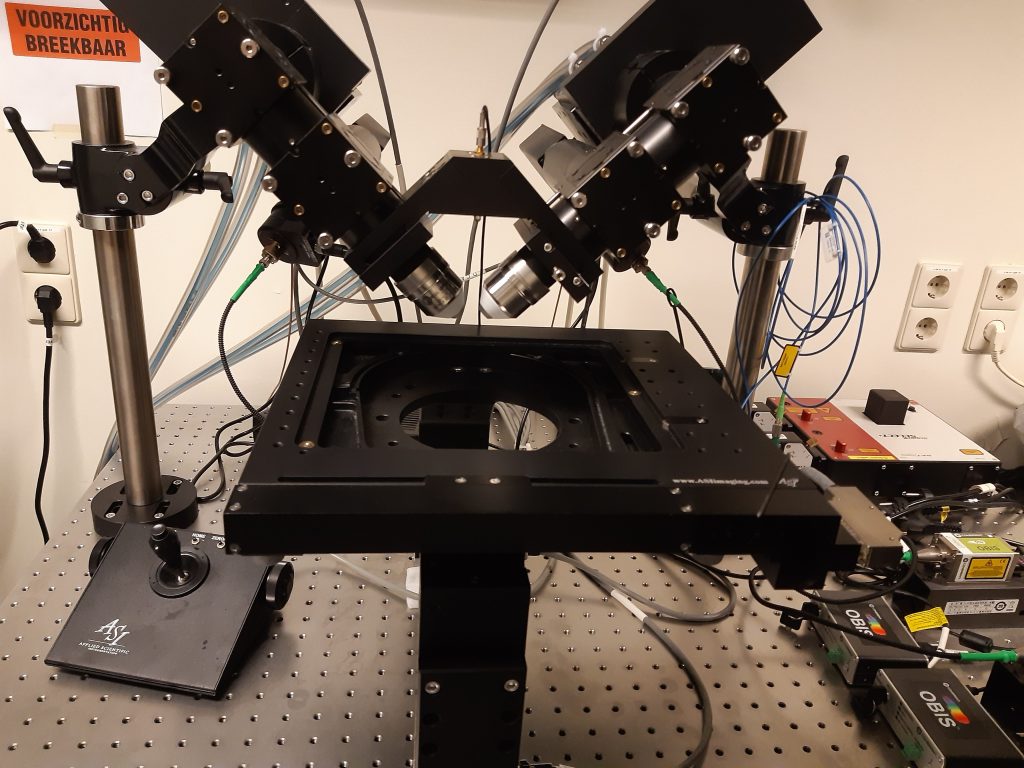
In addition to MRI, we are also routinely investigating post-mortem human brain with light-sheet microscopy. We have set up and built the cleared-tissue dual view Selective Plane Illumination Microscope (ct-dSPIM) to image large-scale optically cleared tissue samples in the imaging lab (for detailed description, see Schueth & Hildebrand, Communications Biology, 2023). The ct-dSPIM was co-developed with Applied Scientific Instrumentation (ASI, Eugen, US) and is derived from the diSPIM light-sheet microscopy (dual view inverted Selective Plane Illumination Microscopy, ASI). We can examine tissue samples up to 5 mm thick and several cm in lateral with so called mosaic scans for fast 3D overviews of entire tissue samples or higher resolution overviews of large ROIs with various speed. This allows us to perform i.e., human brain cell counting or Gleason score grading in the cancer biopsies. To date, we have applied the ct-dSPIM to cleared human brain and prostate cancer samples within the framework of large NWO (Dutch Research Council) and European Consortium (ERC) grants. In the future, our work has the potential to be applied to wide range of tissue types for both fundamental and pre-clinical investigations.
Computational modeling
Computational analysis and modeling is of the utmost importance to use MRI and light microscopy data and test hypotheses and answer questions about human brain connectivity. Investigations in the CBClab not only use the best available methods for image processing and MRI analysis, but also contribute to testing, validating and developing new computational techniques. Computational modeling developments include:
Diffusion MRI tractography and its validation. We have implemented many dMRI tractography methods and compared them for the purpose of macroscale human connectomics (see Bastiani et al., 2012). We have performed extensive validation studies to test to what degree tractography methods produce ex-vivo (on human post-mortem tissue) what is known from anatomy (see Roebroeck et al., 2008) or what is found with histology methods (see Seehaus et al., 2012). These projects also necessitate development of routines for accurate histology micrograph to MRI volume alignment, statistical analysis and 3D visualisation.
Diffusion MRI biophysical compartment modeling. We develop GPU accelerated software for multi-compartment modeling of diffusion MRI data. Such modeling is crucial to make inferences on important neural tissue parameters such as axonal density, dispersion and diameter distributions. We plan release of a flexible python-scriptable toolbox which improves running times of complex model estimations by many times, making estimation more feasible on high resolution dMRI data (Harms et al, OHBM conference, 2014).
Orientational analysis of large FoV micrographs. We have used various techniques to analyse the local orientation of myelinated axons from myelin label light microscopy images. The picture shows 2D structure tensor analysis (red-to-blue bars) of a Gallyas section, compared with diffusion tensor analysis (green boxes) on dMRI data from the same tissue. The diffusion tensor agrees well in areas of single white matter tract direction (middle inset), but less well in areas with multiple directions (bottom) and in gray matter (Seehaus et al., SfN conference, 2013; OHBM conference 2014).
Computational causal modeling of fMRI. We have developed the Granger causality mapping (GCM) method for fMRI, aimed at exploratory region selection for causal modeling (Roebroeck et al., 2005, 2009). We have also contributed to the Dynamic Causal Modeling (DCM) framework, proposing improvements to its neuronal interaction model and hemodynamic model for fMRI (e.g. Havlicek, OHBM conference, 2014). As methodological developments continue in the challenging field of fMRI causal modeling, these two methods are increasingly converging in terms their aims and mathematical underpinnings (Roebroeck et al., 2011, Valdes-Sosa et al, 2011, Stephan & Roebroeck, 2012). Moreover, with the availability of ultra-high field MRI technology and other MRI developments, UHF fMRI at high spatial (< 1mm) and temporal resolution is possible. This allows development of physiologically more realistic microcircuit models in which inter-areal interaction parameters can be estimated from fMRI data (Roebroeck & Goebel, Ch.76 in The Cognitive Neurosciences V, 2014).